Principal component analysis
Also known as PCA
Principle Components Analysis (PCA) is an unsupervised method primary used for dimensionality reduction within machine learning. PCA is calculated via a singular value decomposition (SVD) of the design matrix, or alternatively, by calculating the covariance matrix of the data and performing eigenvalue decomposition on the covariance matrix. The results of PCA provide a low-dimensional picture of the structure of the data and the leading (uncorrelated) latent factors determining variation in the data.
Source: Papers With Code
Image source: Wikipedia
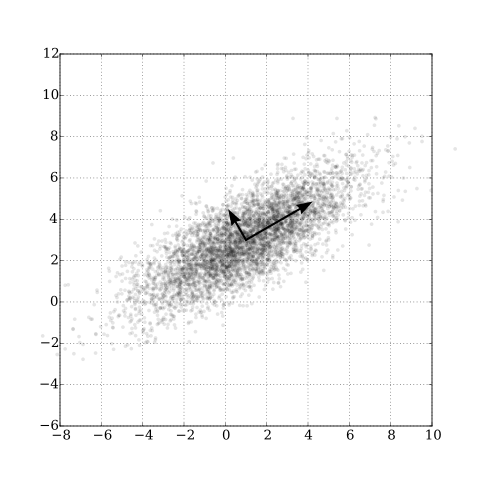
Papers
Date
A Bayesian approach to unsupervised one-shot learning of object categories
Li Fei-Fei • R. Fergus • P. Perona
13 Oct 2003
A Comparative Evaluation of Unsupervised Anomaly Detection Algorithms for Multivariate Data
Markus Goldstein • S. Uchida
19 Apr 2016
Brain-inspired automated visual object discovery and detection
Lichao Chen • Sudhir Singh • Thomas Kailath • Vwani Roychowdhury
30 Sep 2019
Unsupervised Object Discovery: A Comparison
Tinne Tuytelaars • Christoph H. Lampert • Matthew B. Blaschko • Wray Buntine
25 Jul 2009
Unsupervised Object Discovery and Co-Localization by Deep Descriptor Transforming
Xiu-Shen Wei • Chen-Lin Zhang • Jianxin Wu • Chunhua Shen • Zhi-Hua Zhou
20 Jul 2017